Serverless computing is the fastest-growing cloud service model right now, with an annual growth rate of 75%, according to RightScale’s Cloud report. That’s hardly surprising, given the technology’s ability to lower costs, reduce operational complexity, and increase DevOps efficiencies.
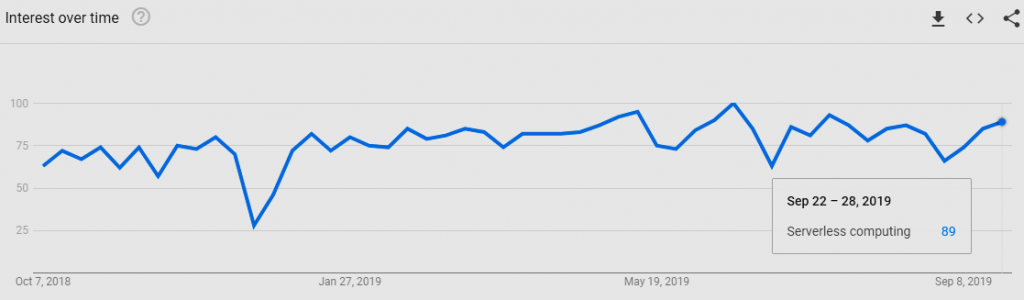
So, as the calendar was poised to turn to a new year, we asked several experts what to expect next from this rising technology. While we received a wide range of answers, everyone agreed that serverless will mature and see even greater adoption rates in 2020.
Why use Serverless computing?
Serverless computing offers a number of advantages over traditional cloud-based or server-centric infrastructure. For many developers, serverless architectures offer greater scalability, more flexibility, and quicker time to release, all at a reduced cost. With serverless architectures, developers do not need to worry about purchasing, provisioning, and managing backend servers. However, serverless computing is not a magic bullet for all web application developers.
What are the advantages of serverless computing?
No server management is necessary
Although ‘serverless’ computing does actually take place on servers, developers never have to deal with the servers. They are managed by the vendor. This can reduce the investment necessary in DevOps, which lowers expenses, and it also frees up developers to create and expand their applications without being constrained by server capacity.
Developers are only charged for the server space they use, reducing cost
As in a ‘pay-as-you-go’ phone plan, developers are only charged for what they use. Code only runs when backend functions are needed by the serverless application, and the code automatically scales up as needed. Provisioning is dynamic, precise, and real-time. Some services are so exact that they break their charges down into 100-millisecond increments. In contrast, in a traditional ‘server-full’ architecture, developers have to project in advance how much server capacity they will need and then purchase that capacity, whether they end up using it or not.
Serverless architectures are inherently scalable
Imagine if the post office could somehow magically add and decommission delivery trucks at will, increasing the size of its fleet as the amount of mail spikes (say, just before Mother’s Day) and decreasing its fleet for times when fewer deliveries are necessary. That’s essentially what serverless applications are able to do.
Applications built with a serverless infrastructure will scale automatically as the user base grows or usage increases. If a function needs to be run in multiple instances, the vendor’s servers will start up, run, and end them as they are needed, often using containers (the functions start up more quickly if they have been run recently – see ‘Performance may be affected’ below). As a result, a serverless application will be able to handle an unusually high number of requests just as well as it can process a single request from a single user. A traditionally structured application with a fixed amount of server space can be overwhelmed by a sudden increase in usage.
Quick deployments and updates are possible
Using a serverless infrastructure, there is no need to upload code to servers or do any backend configuration in order to release a working version of an application. Developers can very quickly upload bits of code and release a new product. They can upload code all at once or one function at a time, since the application is not a single monolithic stack but rather a collection of functions provisioned by the vendor.
This also makes it possible to quickly update, patch, fix, or add new features to an application. It is not necessary to make changes to the whole application; instead, developers can update the application one function at a time.
Code can run closer to the end user, decreasing latency
Because the application is not hosted on an origin server, its code can be run from anywhere. It is therefore possible, depending on the vendor used, to run application functions on servers that are close to the end user. This reduces latency because requests from the user no longer have to travel all the way to an origin server.
What are the disadvantages of serverless computing?
Testing and debugging become more challenging
It is difficult to replicate the serverless environment in order to see how code will actually perform once deployed. Debugging is more complicated because developers do not have visibility into backend processes, and because the application is broken up into separate, smaller functions.
Serverless computing introduces new security concerns
When vendors run the entire backend, it may not be possible to fully vet their security, which can especially be a problem for applications that handle personal or sensitive data.
Because companies are not assigned their own discrete physical servers, serverless providers will often be running code from several of their customers on a single server at any given time. This issue of sharing machinery with other parties is known as ‘multi-tenancy’ – think of several companies trying to lease and work in a single office at the same time. Multi-tenancy can affect application performance and, if the multi-tenant servers are not configured properly, could result in data exposure. Multi-tenancy has little to no impact for networks that sandbox functions correctly and have powerful enough infrastructure.
Serverless architectures are not built for long-running processes
This limits the kinds of applications that can cost-effectively run in a serverless architecture. Because serverless providers charge for the amount of time code is running, it may cost more to run an application with long-running processes in a serverless infrastructure compared to a traditional one.
Performance may be affected
Because it’s not constantly running, serverless code may need to ‘boot up’ when it is used. This startup time may degrade performance. However, if a piece of code is used regularly, the serverless provider will keep it ready to be activated – a request for this ready-to-go code is called a ‘warm start.’ A request for code that hasn’t been used in a while is called a ‘cold start.’
Vendor lock-in is a risk
Allowing a vendor to provide all backend services for an application inevitably increases reliance on that vendor. Setting up a serverless architecture with one vendor can make it difficult to switch vendors if necessary, especially since each vendor offers slightly different features and workflows
Who should use a serverless architecture?
Developers who want to decrease their go-to-market time and build lightweight, flexible applications that can be expanded or updated quickly may benefit greatly from serverless computing.
Serverless architectures will reduce costs for applications that see inconsistent usage, with peak periods alternating with times of little to no traffic. For such applications, purchasing a server or a block of servers that are constantly running and always available, even when unused, may be a waste of resources. A serverless setup will respond instantly when needed and will not incur costs when at rest.
Also, developers who want to push some or all of their application functions close to end users for reduced latency will require at least a partially serverless architecture, since doing so necessitates moving some processes out of the origin server.
When should developers avoid using a serverless architecture?
There are cases when it makes more sense, both from a cost perspective and from a system architecture perspective, to use dedicated servers that are either self-managed or offered as a service. For instance, large applications with a fairly constant, predictable workload may require a traditional setup, and in such cases the traditional setup is probably less expensive. Additionally, it may be prohibitively difficult to migrate legacy applications to a new infrastructure with an entirely different architecture.